Optimizing photovoltaic production with artificial intelligence: more accurate prediction and detection of faults
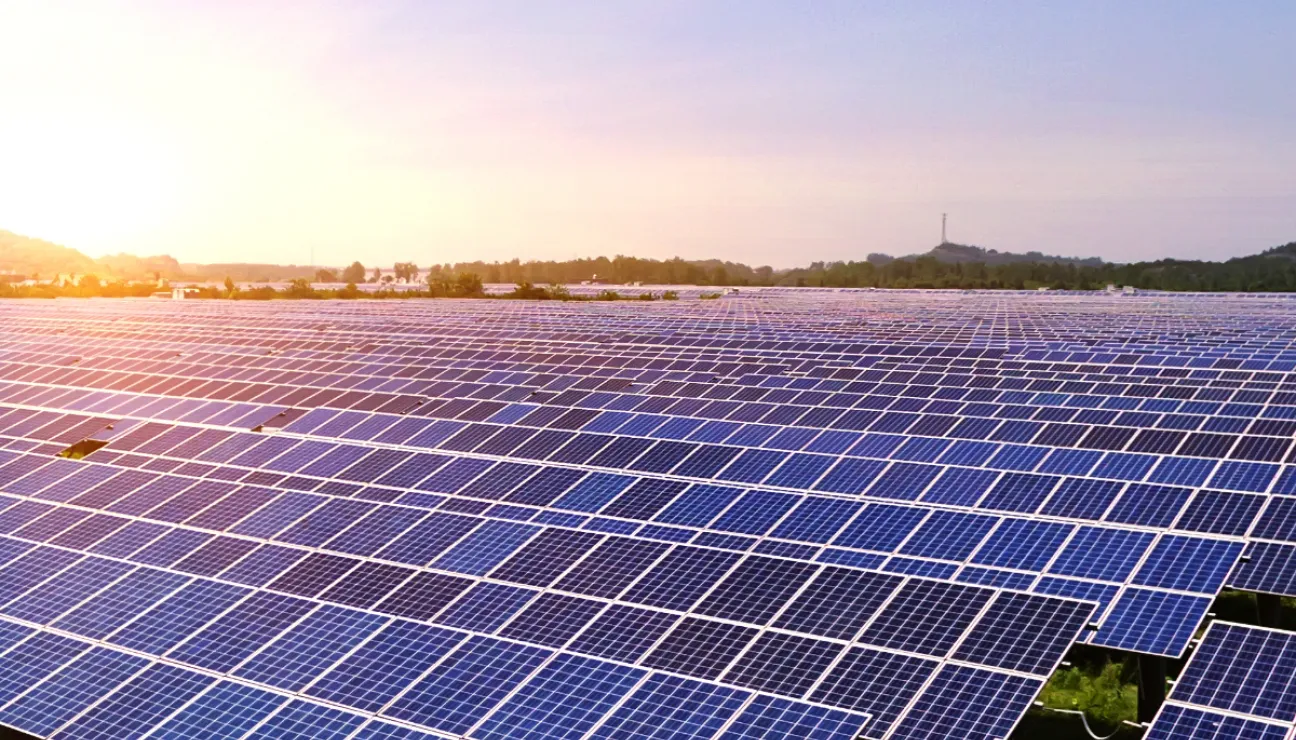
More and more plants are using machine learning tools to improve the accuracy of solar production estimation, optimize the relationship between climate and energy yield, and detect faults in time to reduce economic losses.
Solar energy has grown exponentially in recent years, surpassing 1,600 GW of installed capacity by 2023 and growing at an annual rate of 30%. However, PV plants face operational challenges that can impact their profitability and efficiency such as:
- Inaccurate production estimates due to weather variability.
- Lack of correlation between weather data and energy yield.
- Difficulty in the early detection of failures that can generate economic losses.
Faced with this problem, artificial intelligence (AI) has become the key solution to accurately predict production and anticipate failures in photovoltaic inverters.
How do machine learning & deep learning algorithms work?
Machine learning and deep learning algorithms are non-parametric models that learn from the behavior of historical data. This methodology is suitable for analyzing large volumes of climate, production and operational data. These models can more accurately predict solar power generation, detect patterns in performance and anticipate potential system failures.
Our artificial intelligence models with machine learning are able to relate key data measured at the plant, solar irradiance, ambient and module temperature to energy production. The historical data feeds a machine learning system, which adjusts the predictive models according to the specific conditions of each plant, allowing to detect patterns, optimize performance and continuously improve the accuracy of the system.
Implementation without interruptions
One of the main benefits of this technology is that its implementation does not require production stoppage. Thanks to their integration into the existing infrastructure, AI systems can start operating progressively, analyzing data in real time and optimizing energy generation without affecting plant operations.
In addition, the transition to these intelligent systems enables continuous monitoring and a faster response to possible incidents, minimizing downtime and maximizing the efficiency of PV assets.
The most efficient model: Gradient Boosting
Among the various existing machine learning models, the most efficient model for predicting PV production is Gradient Boosting. This model achieves a balance between accuracy and uncertainty handling capacity, which makes it the best option to improve the operational management of solar plants. Gradient Boosting makes it possible to:
- Reduce prediction errors by progressively adjusting the model.
- Handle large volumes of data with high efficiency.
- Adapt to the specific conditions of each plant.
Why integrate artificial intelligence in your photovoltaic plant?
Thanks to technologies based on artificial intelligence (machine learning and deep learning), photovoltaic plants can optimize their performance, improve operational management and maximize profitability.
If you want to improve the performance of your plant, AI is key. Here are four reasons to incorporate it:
- Increased profitability and adaptation to the energy transition: Artificial intelligence optimizes PV generation by 5% to 10%, resulting in improved consumption and reduced operating costs. This accelerates the return on investment to less than two years, as well as facilitating regulatory compliance and integration with batteries and smart grids.
- Predictive maintenance and cost reduction: AI-based systems detect failures early, avoiding unplanned downtime and reducing operating costs by up to 40% thanks to real-time data analysis. They also optimize maintenance and planning, extending the service life of equipment and minimizing downtime.
- Accurate forecasting and energy optimization: AI models analyze meteorological data to forecast PV production with high accuracy, facilitating grid integration and optimizing energy supply management. In addition, they incorporate 90%-99% confidence intervals to measure uncertainty in predictions, improving consumption planning and optimization.
- Anomaly detection and reduced yield loss: Using artificial vision and drones with thermal imaging, AI identifies faults in degraded panels, inverters and modules, allowing immediate adjustments to minimize losses and reduce the impact on production.
Contact us to learn how to optimize the production of your photovoltaic plant with artificial intelligence.
.jpg)